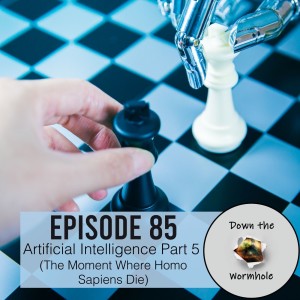
Episode 85
You may be wondering if something is wrong with your podcast feed because this episode is clearly about AI when we already finished that miniseries. Well, that’s my (Zack) fault. I released the wrong episode last time and got us all out of order! Honestly, I’m impressed I made it 85 episodes without messing up the order. This episode is a sort of nice bow on the series. We break down the difference between artificial intelligence, machine learning, and deep learning. We talk about the rights of non-organic beings, whether robots can convert to Judaism, and what our creations teach us about ourselves. We'll also touch on the ethics of data harvesting and whether Google's AlphaGo computer signals the end of homo sapiens as we know it...
Support this podcast on Patreon at https://www.patreon.com/DowntheWormholepodcast
More information at https://www.downthewormhole.com/
produced by Zack Jackson
music by Zack Jackson and Barton Willis
Show Notes
To Read:
1) Alphago deep learning
https://deepmind.com/research/case-studies/alphago-the-story-so-far
2) Alternative reading of Alphago
https://www.wired.com/2016/05/google-alpha-go-ai/
3) Terminator “skynet” character
https://terminator.fandom.com/wiki/Skynet
4) Defense of the skynet character:
https://medium.com/humungus/in-defense-of-skynet-3fd56d04b06f
5) Engineered or Evolved:
https://theconversation.com/evolving-our-way-to-artificial-intelligence-54100
6) Book (don’t read if you’re happy)
https://www.amazon.com/Sparrow-Novel-Book-ebook/dp/B000SEIFGO
7) Song: machine generated, Frank Sinatra covers Britney Spears “toxic”
https://www.youtube.com/watch?v=mbh3VAzrwh8
[the original version: https://www.youtube.com/watch?v=LOZuxwVk7TU ]
8) Book
https://www.amazon.com/Creativity-Code-Art-Innovation-Age/dp/0674988132
Transcript
This transcript was automatically generated by www.otter.ai, and as such contains errors (especially when multiple people are talking). As the AI learns our voices, the transcripts will improve. We hope it is helpful even with the errors.
Zack Jackson 00:00
Hey there, Zack here. You may be wondering if something is wrong with your podcast feed because this episode is clearly about AI when we already finished that mini series. Well, that's, that's my fault. I kind of released the wrong episode last time and got us all out of order. Honestly, I'm impressed. I made it 85 episodes without messing this up. Hooray for me. This episode is sort of nice a bow on the series though. It's a really wonderful episode, we had lots of fun with it. We break down the difference between artificial intelligence machine learning and deep learning. We talk about the rights of non organic beings, whether robots can convert to Judaism and what our creations tell us about ourselves. So without further ado, Let's get this party started. You are listening to down the wormhole podcast exploring the strange and fascinating relationship between science and religion. This week our hosts are
Rachael Jackson 01:05
Rachael Jackson, Rabbi at Agoudas, Israel congregation Hendersonville, North Carolina and if I were stranded on a deserted island with a I would choose the AI computer from Star Trek next generation, Zack Jackson
Zack Jackson 01:21
UCC pasture and Reading Pennsylvania and if I were stranded on a desert island with one AI I would choose Google Assistant because no one in my life knows me better. Now
Adam Pryor 01:41
Adam Pryor, I work at Bethany College in Lindsborg Kansas. If I were stranded on a desert island with an AI. I would choose the mind uploading software from a book called mind scan, which operates as a quasi AI but allows you to then transport your consciousness into artificial bodies in other places,
Ian Binns 02:06
so I'd get the best of both. Ian Binns Associate Professor of elementary science education at UNC Charlotte. And if I had to be stranded on an island with AI, Siri,
Kendra Holt-Moore 02:19
yeah, Siri, Kendra Holt-Moore, PhD candidate, Boston University. And if I was stranded on a desert island with an AI, I think maybe at this point, I would go with our two D two because he just like always survives, you know, he gets into a lot of sticky situations and always comes out alive or always saves people who are in sticky situations and being on a desert island. That's a sticky situation. So
Ian Binns 02:48
RTD I feel like he could probably tap into the midichlorians probably see, so there you go. Maybe we are on right track here.
Zack Jackson 02:57
Thank you, Kendra. You're welcome. Not sure how that's how
Kendra Holt-Moore 03:00
I'm not sure that I agree with you. But you're welcome. Yeah, thank you.
Zack Jackson 03:06
Well, we kind of have a confusion of terms there. Ian's trying to make midichlorians from the Star Wars into artificial intelligence, when I'm pretty sure in canon, their biological. But the terms here that we've been using the past couple of weeks are a little squishy. And maybe we haven't clarified them super well. So since this is the last episode in our series on AI, we're going to kind of clarify some things and give some final thoughts for now we'll probably revisit this topic in the future since this sort of technology is just happening so quickly into our lives. And especially with the rollout of 5g, I mean, that's going to be a whole other conversation about the Internet of Things. And when you have refrigerators and toasters that are as smart as your iPhone is now the world is going to look a lot different in the next couple of years when we will definitely still be podcasting. So we want to answer a couple of questions that we ourselves have had and also tell you that we are going to be adding a q&a segment to the end of every episode. So if you have questions that you want answers if there's something from the episode that was unclear if there is a pressing question you have in your mind if you have any question what so ever that is at least somewhat tangibly tangentially connected to the podcast, then you can leave it on our Facebook page on Twitter, you can email it to admin at down the wormhole comm any way that you can get in touch with us to ask that question. We want to answer it at the end of every episode. So please send that in. But the most pressing question today. What is AI? What is artificial intelligence other than a very sappy movie from the early 2000s Well, we've kind of been using some of these terms interchangeably when we probably shouldn't have. So artificial intelligence is just an overarching term for any machine that acts like a human, or has human like intuition. So your Roomba, that's able to tell that there's a chair leg and moves out of the way, that's artificial intelligence, the computer player in Mario Kart, that's artificial intelligence, the machine that beat the championship go player, that's artificial intelligence. But there's all these different branches of it that we just need to clarify real quick. So like, at the most foundational level, you've anything that has a set of stimulus, and then response is artificial intelligence. So if you're programming something, all it needs to be is a series of if then statements. So if there's a table leg in the way, then move for your Roomba. So an example of this kind of rudimentary level, is all of the battles and Lord of the Rings. You know, when you have like 10s, of 1000s, of orcs, and humans and elves, and all of them coming at each other, that was a computer program called massive, which stands for multiple agents simulation system in virtual environment. One of those really forced acronyms backronym, right. But they're called. So what that was, was each and every one of those characters had its own set of physics, and its owns awareness of what was happening around it. And they were told, attack. And if you encounter this, then this happens and encountered that, then that happens. And so you do that on a large enough scale. And you could have 10s, of 1000s, of orcs and humans and elephants, and all kinds of things fighting each other. And the computer is determining each and every outcome based on a set of, of commands. Actually, in early renders, the humans kept on losing. And so they had to keep modifying it in in a couple of cases, when the olifants came over, over the side, the humans retreated. And they kept giving up because it was too hard, and which is such a human thing to do. So a lot of the special effects that you see are done like this. So then one step in to that, nested within that, if you think of it, like a series of nested eggs, is machine learning. And this is artificial intelligence that gets smarter, the more you use it. Basic machine learning has been around for a long time, if you've ever had to prove your human by answering a CAPTCHA, you know, show all of this, but click on all the squares that have a crosswalk. That's you are actually training an artificial intelligence by doing that, you are helping them to learn how to identify crosswalks so that that information can then be used by machines in the future to for some other purpose. This level of machine learning requires human input to say to it, this is a crosswalk, this is not a crosswalk, and then the artificial intelligence then takes it apart pixel by pixel. And enough input from humans will teach it what to look for and what not to look for. And then once it has a grasp on it, it can start doing it itself. And the more that it does it, and is confirmed that that's the right thing, the better it gets. And so this process can take months and months and months of of teaching this machine, the way that you would teach a child that this is this that is not that my local recycling plant has this incredible system driven by machine learning where it's got all these cameras and sensors, and it can tell different types of plastic. And then it can sort them with machine arms and with all kinds of fun things that they can do to then sort the plastic faster than you could with humans, which then means that it can be more profitable, and they can recycle more and be less wasteful. So like, that's pretty great. And the longer they use that system, the smarter it gets, the better it gets at determining different types of plastics and metals and whatnot. So that's great. I'm totally into that. It takes a lot of human input, though, in order to teach it. So then, the next level of nested egg of artificial intelligence is what's called Deep learning. And this is the future. This is actually the present. If you've interacted with google assistant or Siri or any kind of customer service You have likely interacted with deep learning. Deep Learning does what machine learning does, but it doesn't require humans to, to teach it first. So an example of this is Google's AlphaGo, which is a program, the supercomputer that was created to beat a human go player, the ancient Chinese game. And the thing about go is that it has, I think it's I read somewhere, it's like a million times more potential know, the difference between the potential moves in chess and the potential moves in go is more than all of the atoms in the entire known universe, there is so much, so many different moves, you can make an any different point. Whereas chess players are thinking about potential moves, a lot of times, like the best go, players are just feeling it, it's intuition for them. And so this is kind of the gold standard for teaching artificial intelligence to beat humans and to think like humans. So what they did was they they took this AI that they developed, and they had it watched 100,000 games of go. And so it's analyzing every single pixel of this, and putting it through its neural network and breaking it all apart and deciphering the rules itself. And then after 100,000 times of watching it, it had learned the rules on its own, because like it knew that this person one and this person last, and this person did this, and this criteria was met. And so it learned the rules itself, nobody had to teach it, it learned it itself. And then they set it to play against itself 30 million times, it's simulated 30 million games against an older version of itself. So it would learn for a while to get a little bit better, and then face its previous self, and then that version would get a little better, and then it would face the version before it. So it's always facing a version that it can beat, but just by a little bit. And after 30 million times, it faced the number one champion go player in the world and beat it beat him in 2017. So those, what, five years, four or five years ago, that it is for this happened for Yes, thank you for thank you for math, we're not yet in 2022. So in this case, it took no human interaction for this thing to learn and then to be able to thrive. They've got these playing old, old games from the 70s and 80s. It learned how to beat Space Invaders overnight, and just defeat the whole game without teaching it any other rules. It just lost enough times to know how to avoid things and how to time things and all of that. What this requires, however, as opposed to machine learning, and AI, in the simpler senses, is a ton of data. So this required 10,000 100,000 games of go, it had to watch in order to figure out the rules of the game. So if we have a ton of computing power, and a ton of data, then we can create systems that can teach itself how to best optimize itself, and then is able to find avenues that the human mind is not able to find. So this is where we are now, which obviously there are some issues with because if you're not being very careful about the data that you're feeding it, then the output is going to end up being potentially skewed. On the other hand, because we're not intentional about which we're not giving it specific data sets, we're giving it everything, it's able to make patterns that maybe we wouldn't have otherwise, it's already proven to be so effective at locating cancer cells of predicting stock movements, which is causing all kinds of issues, was one of the reasons why the GameStop stock went crazy was because humans out out, bought the algorithm and then crashed the stock market basically for a while. It's also one of the things that's contributed to the crazy gerrymandering that we have now, because the computer is able to run every simulation of every election we've ever had against population data and then determine the ideal gerrymandered district for how population growth is going to go and immigration and all of that to make sure that their people stay in power. So like there's a lot of potential in this kind of technology. To be amazing, like, imagine a shirt that was able to sense your body temperature and your sweat, and the temperature and the forecast and then to be able to adjust its fit, depending on your particular comfort level. So like the person who's like, I'm always cold, they don't have to be always cold anymore, because your shirt knows you, and it loves you, and it wants you to be warm and comfortable. But at the same time, there's all kinds of potential issues where it'll still smell bad. It'll say, Well,
Rachael Jackson 15:33
I was thinking about how to wash your shirt like that,
Kendra Holt-Moore 15:35
unless it's merino wool. Unless it's what will Marina will?
Zack Jackson 15:40
Sorry, is that fancy? Well, which I don't know? Well, yeah. Well, resistant, very, I'm a simple man,
Kendra Holt-Moore 15:45
I've been preparing for backpacking this summer. And that's. So an example of issues with big data.
Zack Jackson 15:58
Okay, so all of these companies that are trying to teach computers how to talk and to communicate with humans, naturally use these huge language models, where they take communications from spoken communications from television, and radio, and podcasts even and, and they take written communications from websites and emails and text messages. And they create models of the English language, let's say, and, and then are able to do the sort of predictive texts that you see, or Google is able to translate websites just in a snap. There are some concerns. And one of the couple of months ago, the head of Google's ethics team co authored a paper that her name is timnit gebru. And she co authored a paper for conference, questioning some of the ethics behind this because if you're just gathering data, just haphazardly, because you need a ton of data to make this kind of system work. If you're just gathering it from everything, and you know, Google, the Google algorithm has access to every google doc and every email and everything that you have ever used on their system they have, that algorithm can access whenever it wants to, if you're just taking all of that in order to learn the English language, and you're not taking into consideration prejudices and violence and the awful things that make humans human, then you have the potential to create an algorithm that will say have implicit bias built into it the way that humans have it built into us, because it's just the air we breathe. And so she questioned in this paper, that not specifically calling out Google but IBM and Microsoft and everyone else that's doing it. And Google demanded that she retract her name from it. And when she refused, because she wanted to know who exactly it was that was censoring her, and why they were censoring her and what she's allowed to say in her position and what she's not. They fired her, though, they said they told everyone that she quit. Yeah. And she has said many times, I did not quit you, I say you fired me, I don't work there anymore. And I didn't quit. That means you fired me. And they still kind of refused to acknowledge that they fired her over questioning this kind of potential issue with data. And so that's not a good look for Google. Because they are, this is kind of their business. They're in the business now of big data and predictive tax, and they need to be on top of it. And it's a threat to their bottom line if if people start questioning the ethics of it, and they start opting out of that. So that raises all kinds of issues about oversight about trusting capitalist systems to self correct for morality, versus government systems for that as well. The potential for a self learning machine to become smarter than us and realize it doesn't need us or the potential for it to fill in all of the gaps of our weaknesses and help to create a utopian society. It seems like the future is wide open and we are at a transitional tipping point right now. And that is my long intro into all things AI and machine learning and deep learning and the fear and the optimism of the future.
Ian Binns 20:08
So can I ask a question? No, you just don't want to do anymore. All of the it sounds like even, you know, maybe from the initial beginning of things that is still depends on human input in some way to to then get things going. Is that accurate?
Zack Jackson 20:28
But like humans have to create it,
Ian Binns 20:31
either and create and then started on the learning process until they started doing it on its own, like from the the initial outset. Like it doesn't start on its own right.
Rachael Jackson 20:44
I don't think anything does doesn't I mean, started jumping? No. Doesn't does anything start on its own? Or like, your father? Did your children start on their own? That that,
Ian Binns 20:57
that that topic will come up in a later episode, Rachel, you know, it is. Not that they
Rachael Jackson 21:09
came into existence on their own, but that, as you're raising them, they don't just know what you expect of them. Right. And so for me, I don't know why we would expect anything different at this point until AI creates its own AI. And perhaps that's because I was watching Agents of SHIELD where AI is creating AI and I just want to cry. And how the sort of sci fi horror that comes with that. But I think, for me, the analogy is absolutely each one of those things, right? It's very much a parent child relationship for for those circles within circles, how much input is someone giving it? Or how much are you just being taught to do on your own, and a whole lot of garbage in garbage out?
Ian Binns 21:55
But I guess what I'm saying is, is that I guess, where I'm trying to go with this is that being that it always like, even if AI gets advanced enough to the point where it can create its own AI, the initial AI still had to be depend on human input to get going.
Rachael Jackson 22:14
Yes, all of our biases, all of our motives, all of our garbage, is my point is going to be a part of it until somebody teaches it or trains it to remove it in my opinion.
Adam Pryor 22:25
But I think that's what's terrifying about deep learning. Is that it Yeah, it's not reliant on that. Like, so this is what this is what's notable about the AlphaGo experiment, right? So this this experiment where they're playing go, right, so like, it's not just that AlphaGo beat Lisa doll, it beat Lisa doll three times in a row. And the second time it beat Lisa doll, it made a move that no go player thought was a good move. He made a totally novel move, it's in fact changed the way that go players play by analyzing this game. So okay, it took it. So like, mathematicians will describe this in terms of like a local maximum. Right? Go players had reached a local maximum in the landscape of potential go moves that one could make. And everyone thought that was the highest point AlphaGo utterly creatively changed the strategy by which the game is played. It found a new maximum in the landscape of possibilities. Right, that that's the piece of deep learning that it was not relying on human beings. Okay. It was not taught to do it. It was a completely novel structure structure that it came up with vinted on its own.
Ian Binns 23:46
Yes. all on its own. Like it. This was not from analysis. Nope. of Oh, I, you know, the thing was able to remember a move in the past this was told this was a totally novel move. That's really interesting,
Zack Jackson 23:59
right? That's what the old ones like Watson, when they're playing chess, they would look at the board and all possible moves, and then would plan ahead for all possible moves like three times in the future. That is kind of rudimentary machine learning. This one got creative.
Kendra Holt-Moore 24:17
But that creativity, I mean, I don't know that that creativity still is based on what the AI has learned from humans. It's not that the novel move. Yeah, maybe like humans had never done that before. But it's like a process of elimination of what didn't work that humans had done. And so the novelty is still sort of playing in opposition to human.
Adam Pryor 24:46
No, it's because that would be so that would be that would be a machine learning instance, right. That would be where there's a set algorithm, it analyzes all of the potential moves that would be available and then chooses the best one. Right.
Kendra Holt-Moore 25:01
But what is the best case one is one that was never chosen.
Adam Pryor 25:04
But in this case, it's not working that way. It's modifying the algorithm.
Ian Binns 25:08
And that's where the deep learning comes into play.
Kendra Holt-Moore 25:12
Okay, yes. That's creepy. Yeah, okay. Well, that that kind of.
Adam Pryor 25:18
I mean, it is still like children, though. I mean, like, I think that's still like a good like an out like, right, like, but there comes a point, right? Where those children start making decisions on their own. Right? utterly independent of the places from which they came. Deep Learning is rapidly approaching that place, and if not already crossing over into it.
Rachael Jackson 25:38
And just like with children, or, you know, I'm not I'm not saying children as in a particular age set, but the parent child relationship, looking at them going, you know, you can have that moment of like, wow, where did that come from? That I'm now seeing you. It's no longer what I'm teaching you and you're a being back to me. It's like this was you. And then there can be that moment of like, Oh, my God, what is this? Like, what did I create? And where is this coming from? Like, the, the parents of psychopaths, like I did not create that. They did that all on their own. So I, again, I really like the analogy that a where we are with AI and deep learning and all the concentric circles is still really a parent child relationship.
Zack Jackson 26:27
This has this has a very Genesis three feel to it. Yeah, we're like, God creates these creatures that have advanced knowledge and gives them a set of of do this, don't do that. But I'm gonna let you go and learn on your own. And then
Rachael Jackson 26:43
I want to be God to let me eat this apple.
Ian Binns 26:46
That does make me think about Skynet from Terminator. And when you really think about it right there, they wanted to pull the plug on Skynet, because they realized this guy that was learning on its own, and becoming better, like learning faster and becoming dangerous to humans. And so they tried to pull the plug. But by then it was too late.
Adam Pryor 27:06
I mean, like, this is the thing to me. Right? Like, Skynet is like the, the, like the best example of this, right? Because the only thing human beings have going for us is that we're better at structuring context. Right? We are never going to win the like data processing game. Computers have beat us out on that for a long, long, long time. And it's only the distance is only growing further. Right? It's, it's when you reach this point with deep learning that algorithmic systems produce better analyses of context than humans do. Which is really what Skynet does, right? Like it's it's gathering all this data, and then suddenly can produce a better vision. Right, of what the original outcome that it sought, is then what? Humans you know, what our key was, okay?
Ian Binns 28:04
Or like Vicky from iRobot? Ah, yeah, that's a good example. That would be a really good example of realizing to Vicki, I think there's even a part in that film. Where, Vic, like, when they realize that the one who's been doing this bad stuff the whole time was Vicki. And she talks about, I think, something like realizing that humans were unable to do these things for themselves. And so Vicki felt like it was time for me to take over. Because humans are too dangerous, and all that kind of stuff. And then it just, yeah. All the problems that exists because of humans. So let me take over and I'll fix everything by killing.
Kendra Holt-Moore 28:43
Nice. But yeah, but also rational. Yeah, yeah. Oh, no, just this conversation is making me rethink. Or maybe not rethink, but I'm just wondering about, like, the usefulness of this frame, like comparative framework that someone published an article, I think it was in Scientific American, or somewhere, I can look and send that to Rachel, but he's a scientist and was talking about, like, the way to think about AI. It's something that has evolved or like, is evolved, rather than engineered. And that, you know, I think, sort of like colloquially, especially for people who aren't scientists making robots, we think our like gut reaction is like, oh, someone made that someone like engineered this robot to perform this function. And that that, you know, Like, on some level is true, but it's not actually the end game of AI in like the grand scheme of, like what humans are trying to do with AI, it given our conversation about, you know, the, the, the goals of like being better and better with each generation of AI. Like they're vastly outpacing a lot of our, like human abilities already. And that's just like continuing to happen at greater degrees. And so there is an evolution, like, have these things. It's always been that way. But I guess like the the main point of that comparison between, like evolution versus engineering is that thinking about AI, in terms of evolution, is more like thinking about human intelligence, and, like the evolution of human ness. And that there's something very similar, maybe, like, eerily so that robots are, you know, that's like what we're doing. And I think that the example that I think it was Rachel, who a few minutes ago, like, brought up the kind of analogy to like a child, or like that parent child relationship that, you know, there is like a starting like a baseline of what AI is and what it knows. But if you have to have like mechanisms in place for it to, to grow and learn and that simple Engineering Task, where you're creating a thing to solve issue a, there's not an an implied, like, growth are like evolution factor in a simple Engineering Task. I mean, it can be but not always. And so I just, I think that's really interesting, because I, I just, like, evolution. Like, I guess I'm struggling always with, like, what the morality of like, what, what are the implications of this? the morality of AI, because I'm, like, thinking about the evolution of AI. And in that one day, it's just gonna like, or, you know, apparently, I've been misunderstanding robots. And it's already like creatively coming up with novel solutions. that evolution is kind of this like, opportunistic, amoral system. I guess that's how I think about it. And so whether you're talking about AI in terms of engineering, or evolution, you still have these like social political issues. And that doesn't go away. And it's a little concerning, because I think that, like some scientists will see themselves as outside of that problem. Like, science for the sake of science, seeking the truth, uncovering whatever is before us, whether that's good or bad. And there's something really exciting about that ideal, but like, it's out of it, we don't like live in a vacuum, and you can't, you can't, like seek that kind of big t truth without considering these other contextual factors have implications for harm and things like that, which is, you know, what we've been talking about. So anyway, that, the other thing I wanted to say is that this sort of relates to I think, one of the first episodes we recorded about personhood, and just thinking about the kinds of AI that are so much like, us, I'm just wondering about future conversations where, you know, like, we talk a lot now in the 21st century about human rights, and the importance of human rights. And, you know, we're there are a lot of people who always have but, you know, now these groups, you know, look in different forms of like, animal rights, and, you know, like, hardcore, like veganism and things that are trying to, like, bring animals into the picture and, you know, reevaluate, especially the ways that the West treats nature. And I just am really curious about how robots are gonna make us reconsider even the value of like, human or animal this and like, what will be our big common denominator? Is it is it personhood? Or is it something else? Because personhood is, I think can include robots, but it's still kind of this like weird, a morphus category and human rights is just something that I think especially for like, liberals is like this big like, that's the idea. We want everyone to like, benefit from human rights. And, you know, we want to like not torture animals too. And just like how will that conversation, which is like general and really common in a lot of our circles? How will that change with those smart robots all around us?
Rachael Jackson 35:41
Zach, I want to make sure that you had some time.
Zack Jackson 35:45
I had the first 20 minutes. I was thinking the same thing about everyone else.
Ian Binns 35:58
And can we go back to the AlphaGo thing real quick? Just come just curious. So I was I found a wired article about it, right? And started thumbing through and goes back to what you're saying, Adam, that that move showed that, you know, his creativity that was able to do something that's never been done before on its own. And right here, it talks about this article I'm Reading in Wired Magazine. That particular move wasn't the moment where the machines began their rise to power over our lesser minds. That move was the moment machines and humanity finally began to evolve together.
Adam Pryor 36:37
Right, so this is that like, I mean, I think kendras thing about like, engineering versus evolution for thinking about this is really critical, like, because we do think of machines as engineered objects. Right? And that is not what is happening at this point, right? Like, what I think is like, most interesting, terrifying sort of like, is strategically helpful, potentially, about the way algorithmic learning occurs, which is really like, also, I keep using the phrase algorithmic learning, you could substitute deep learning, right? All deep learning is a form of algorithmic learning, at this point, so what's like both terrifying and potentially helpful about over an algorithmic learning is this place that it has reached, where it allows a new context for local systems to be discovered. It opens up a new way of seeing the data that sits within a given field field of analysis. Now, at least in my area of the world, academically, we might call that meaning formation. And that I think is the sort of like that wired article is I think, Reading AlphaGo, Reading the situation that occurred is AlphaGo, forming new meaning out of this particular landscape of the game. Now, is that what happened? I am a little more suspect, right? Like there's a level of intentionality and consciousness being projected. right on to that situation in order to say that machines and humans are learning together right now, are they evolving together? Right? is are we suddenly in an age where this sort of algorithmic, algorithmic deep learning means humans engage their environment in ways that previously were totally unimaginable? Yes, I'm on board with that. Like, I I'll point to this moment with AlphaGo. And say, like, that may be the moment where Homo sapiens die. If we looked at this millions and millions and millions of years from now, like that might be the demise of our species.
Ian Binns 39:24
So happy right now. I don't think that's a bad thing.
Kendra Holt-Moore 39:31
I'm not ready to die.
Ian Binns 39:34
I like my I like our species, and that I'm still alive.
Adam Pryor 39:37
I mean, the Neanderthals like their species to
Ian Binns 39:41
good fairpoint.
Rachael Jackson 39:48
You know, that's where I was thinking about this, you know, if we, if we really toy with this idea of evolution, and AI, we don't there's past 70 or so. The activity in my head, right engineering means that we are actively doing something and evolution is there's sort of a passiveness that it's that it is happening, or happens like we you just said the Neanderthals like themselves too. And yet they're not here either. And part of that is not the evolution of them, but the evolution of us. And then we killed them.
Zack Jackson 40:23
Right, right. absorb them I'm part Neanderthal, yes.
Rachael Jackson 40:30
Two 3% or something like that, right? Like, absorbed kills those things? Perhaps those things, sports, all those things like people that those things like those changes, I'm not that harsh. Right? Resistance is futile, you understand this? Right? So maybe if we initially engineered machines, machine learnings that then has with our evolution and our engineering, we're sort of making that deep learning turning into ai ai, then over X number of hundreds of years then evolves beyond where we started from. And if that then is our demise, then perhaps isn't that our homosapiens evolution? That, that we have all these branches, and it's not necessarily a fatalistic perspective, but it is much more of a geological time perspective, right, human Homo sapiens have have been around for a blink of an eye.
Adam Pryor 41:40
Not nearly as long as homo Neanderthals were around.
Rachael Jackson 41:42
Exactly. Exactly. And, and who knows how many more iterations before that, that we just don't even know about? And how long they were around? And why why do we think that us human beings are so much more specific and special that we would get to last? You know, 160 million years like dinosaurs did? In a Why? I just don't see I don't I don't have an issue with Homo sapiens no longer existing. Again, not a deathwish not saying that I want us to die now. But but recognizing that in the grand scheme of things of things on this planet, if that's if that's where we're going to go through our own evolution, then that's, that's an okay thing. It's not our demise. Like Adam is saying it's
Adam Pryor 42:38
that no, let's let's be clear, it is still our demise. No, no, I don't want to let you off the hook. That easy. Wait, there's there's demise there. There may be progress. Also. I'm okay with that. But it is still the demise of Homo sapiens.
Ian Binns 42:55
As we know it now. Yes. Okay. Great.
Kendra Holt-Moore 43:00
Thank you, demise, evolution, what's the difference? Tato. But, so to me, like, what's interesting about this,
Rachael Jackson 43:09
maybe we'll be together. Sorry, Adam. Or like, maybe it will be together?
Adam Pryor 43:13
I don't think that's gonna go well, for the like, like, not as fast thinking homosapiens we just say that hasn't traditionally gone real well, for those species, but
Zack Jackson 43:22
think about it an excellent pet way to be.
Adam Pryor 43:28
Have you read the sparrow? No. Okay. Yeah, you need to read the sparrow. And think about that before you decide you want to be a pet. I will just tell you, if you haven't read the sparrow, it is Jesuits in space. It's worth Reading.
Rachael Jackson 43:45
Is this just making sure. Mary Doria Russell, Mary Doria Russell,
Adam Pryor 43:50
yeah. Because it's brilliant. It's a brilliant book. Also, please don't read it. When you're feeling happy.
Rachael Jackson 43:57
You will not feel happy at the end,
Adam Pryor 43:58
you will not feel happy at the end of this book in any way, shape, or form. But all told, even if it is the demise of Homo sapiens, which I think it is, or, you know, a lovely vision of progress where Homo sapiens now live in a utopia with these machines. And we're not just pets. I look at this though. And I do go like, I feel like that's really challenging for religious traditions. Like I think most religious traditions have a sense of the sacredness of the human. And that gets translated to Homo sapiens. Very, very specifically. And, and I think this is sort of like an interesting place where I really do think deep learning is starting to push a boundary for religious traditions to rethink themselves. Maybe not all, but I'll at least speak from my right, like, Lutheran Christian version of this right. It's really hard for me to square any kind of eschatology that we've usually talked about in those traditions, with a vision of Homo sapiens not being permanent fixtures until the Rapture. Right? That's, that's pretty hard to square. It's, it gets kind of harder for me to sort of do a usual Christology. where, you know, Jesus Christ shows up at this very particular point in time, if Homo sapiens aren't going to be the last species of human beings on this planet. I mean, I think there are ways to do it. But but it's a, there are some creative theological questions that I think really emerge from taking this idea seriously.
Rachael Jackson 45:38
If you don't have those two things, though, right, because only some religions have those two things. Would it? Would it work?
Adam Pryor 45:49
Well, what I would say is, like, I wonder are would other religious traditions have their own questions that they have to ask in the face of this? I don't, I don't think all all of them ask the same question by any stretch of imagination, like
Rachael Jackson 45:59
I think about, from my, my corner of the world, right? I'm thinking that in Judaism, what what is the ultimate goal for for the existence of humans or the existence of Jews or the exit, right? Like what what is happening next, not the rapture, not right a second coming up. A Messianic age figure that says the world is now fully repaired, and it will continue to exist in a place of wholeness and peace. It allows for anybody to participate in that. In fact, it it requires anybody and everybody to participate. And if you were at one point, a righteous person, or specifically a righteous Jew, you then get to return in that in that Messianic age, and sort of heaven on earth, right? gun aid in the Garden of Eden, comes once more for us to live happily ever after. And there's no problem with Well, what if it's not me?
Adam Pryor 47:12
Well, but I think maybe then, so as I'm listening to you, right, like the question that would start to emerge for me in my like, philosophy of religion sort of side of things would be like, okay, so can there be a righteous Jew? That's not a homosapien? It feels like there would be internal debate about that.
Rachael Jackson 47:30
I don't actually know. I mean, only because Jews debate about everything. I mean, right. But beyond that question, my gut reaction is, why not? If we're not already limiting ourselves to dues, then we're opening ourselves to everyone. And if we're opening ourselves to everyone, then why couldn't that everyone include an AI? Maybe I'm just optimistic and positive. I don't know. Maybe I'm just in the right religion. I don't know that either.
Adam Pryor 48:27
There's nothing that brings my heart more joy than just hearing you say maybe I'm in the right religion. I'm gonna hold on to that for a while. I just I just want you to know.
Ian Binns 48:42
I say you're always optimistic to
Rachael Jackson 48:45
heavy stuff.
Adam Pryor 48:47
I would bet that there are scholars though, in different religious traditions who, who would be able to say like, there are questions in our questions or propositions or concepts within our tradition that have to be rethought in light of homosapiens not being a permanent feature into the far future.
Kendra Holt-Moore 49:10
Yeah, I think like everything that you're saying, Adam makes a lot of sense. But I I also think there's a version of like Christian eschatology and Christology that on the one hand, you're right, like people will have issues with the, you know, homosapien piece, but there's another side of that where I feel very similar to what Rachel saying, I'm like, yeah, Christians will be fine with it, and they adapt and make up stuff all the time for that's why I have a job. Yeah, so I just think, you know, it'll be like the same as it's always been, like, more hardcore conservative interpretations will maybe be more troubled by this, but I think Christianity has also always done a version of like very liberal and open and like metaphorical interpretation that definitely can survive without homosapiens. However we may feel about that.
Adam Pryor 50:18
Yeah, I mean, I think that would be the case. I, I do think, though, that you end up with a I'm gonna I'm gonna go with this since you know, Rachel's in the right religion, right. I think there are winners and losers out of these, like traditions as a result, like there are. Look, we can use the evolutionary example, right? There are evolutionary streams of various religious traditions that get closed off if you start taking this seriously.
Ian Binns 50:47
Well, so what I'm really curious about I keep coming back to this AlphaGo thing, like, I mean, if you really think about it, that is really amazing. This happened.
Kendra Holt-Moore 51:01
Right, right. Like, it's how to, like sign up for the go championship or something like,
Ian Binns 51:09
no, no. I've never played the game. So I would probably do pretty badly. But no, my point is, is that so this this article that I have from wired magazines, like 2016. So what's happened since then? Like if this was kind of a pivotal moment, in machine learning, and deep thinking, what I mean, Adam, are you aware of anything like that, since then has happened? That's,
Adam Pryor 51:44
well, like more like, Holy moly, I can't believe that happened. So it's interesting to me that like, after this moment, my understanding is that the deep mind team, like so the folks who did Watson, the folks who did AlphaGo, like, they have shifted on to more substantial issues. Things like climate change analysis, right, very specific, like, like, targeting this type of deep learning to specific problems. So like Zack mentioned, like at the beginning, like cancer, cancer Reading, right, so I'm training algorithms to be better interpreters of MRI scans, and CAT scans, then human technicians, right, that's an active project going on at a couple of medical schools, you start to see these very, they tend to be like highly targeted problems right now. In terms of stuff that I'm thinking about, there are some like creativity ones. I taught a an interdisciplinary class with a math professor on this. And Charles Deuce toi, wrote a book called the creativity code that covers like, some of the like, latest things, and he starts like with AlphaGo, and then works on other places. And the book is framed around, like, will we reach a point where deep learning is more creative about mathematics than mathematicians are? is like a sort of, like, problematizing question. But there's some really interesting examples along the way of, you know, like the action Jackson, right, which is a machine painting system. Some other music is a big area, right? Where there are good AI projects working to do composition work.
53:47
Cool. So.
Adam Pryor 53:50
So on the one hand, there are some like kind of like, pet projects in the creativity realm. There, there are a series of like, I think, specific medical and environmental technologies, where you're seeing this used pretty robustly. There was a good piece in The New York Times Magazine on where AI, particularly deep learning systems will end up replacing a variety of middle class jobs. Actually, there was the final one of the final assignments for the students in the class was when their job that they want to pursue be replaced by AI in their lifetime.
Kendra Holt-Moore 54:29
That's a great assignment.
Adam Pryor 54:32
Most of them said probably, and that, you know, that, you know, ultimately, there's something about their humaneness that would prevent them from losing their jobs specifically.
Rachael Jackson 54:43
We talked a little bit about that, I think in a in a previous episode to where we say like, some of us like, our jobs are secure. My jobs not going anywhere.
Adam Pryor 54:54
But mine definitely will go away. I mean, I'd be shocked, actually. Yeah. Part of it will be part certainly part of it would.
Rachael Jackson 55:02
Yeah. One of the things that I just wanted to sort of end on that we've been dancing around but not really talking about is our human relationship to AI. In terms of what will our relationship be, if we're looking at relational or transactional? Those are sort of the buzzwords these days for how to how to be inside a community like are you treating? Is your community? Is your place of worship transactional? Are you fee for service? Or are you relational, and that's how you get people to stay members. Or to stay engaged, not just a membership, because it goes much more beyond those buzzwords of the last half decade. And for me, it goes further back to Martin boober. And the idea of, not just where is God, but where are we, and how are we using the ideas of AI it or I thou relationships, and for boober boober said that we can have an I it relationship with another human being and most of us do almost all the time. Right? The person that at the grocery store, the person the bank, Teller, any delivery service, even friends, even rather acquaintances, and co workers, most of them are I it relationships, very basic, based in transaction I will do for you only because you do for me. And that's sort of how we just can live our life and the antithesis to that is the I thou relationship, the ICU is you and want to be in relationship with new, not because of what you can do for me. And that is that can be true for every person. And also with things you can have an i thou relationship with a tree. And I love that one like I guess I have, I haven't I have an i thou relationship with my tree. And if we take that concept, how right this is, this is sort of my hopeful, optimistic view of where we can go with this, no matter what it is that we're talking about. If it's a deep learning, if it's an AI, if it's if it's, if it's Rosie, if it's your Roomba, you can have the I thou relationship with it, with the object with the creature with this, this something in front of you, and you're better for it as as is that thing. And wherever, wherever we ended up going with it. That's that's the perspective. And that, for me is what I can contribute to these relationships. What my perspective and how I perceive the thing across from me, I'm smiling.
Adam Pryor 57:55
So so I want to know, do you do you say thank you to Siri or Google or Alexa? When when you ask it questions, almost always,
Rachael Jackson 58:04
and it changes that I have I have Alexa. And it changes the sound of its voice. And it'll give me two or three phrases back such as you betcha. And it's like this really fun. Is this really fun statement of like, Oh, you're welcome. Now, just FYI, if you say I love you, it does not say I love you back. It says, you know, like, thank you, which is really smart emotional learning. So you don't find to get that attachment to this object. So that I believe is the programmer is because it's not yet capable of doing that. But maybe we'll get to a point of how and Eureka, right, the TV show Eureka where he's buried to a house.
Adam Pryor 58:47
So I I will say I tell Google thank you all the time. Whenever whenever I asked Google something. Um, but what it did make me recognize is that I don't say thank you to my cats. As we talked about, I though I had relationships,
Rachael Jackson 59:05
but but you don't like your cats. You have until your cats you kind of hate them.
Adam Pryor 59:08
No. So. So this is the like, but when we ask, like, Can we have a closer relationship with machine learning, deep learning than we do with other creatures? You right? Like I don't even have to go all the way to a tree. I can just go to the cats in my house. Right, right. Like any when they get up on the counter. The thing I shout at them is nobody loves you. Right? So like, I mean, I don't shout nobody loves you Google. I say thank you.
Rachael Jackson 59:33
You have a deeper relationship with your Google than you do with your cat. Yeah, I think that's a perfect example. You monster.
Zack Jackson 59:45
This has been Episode 85 of the down the wormhole podcast. Thank you for coming on this journey with us and especially to all you who have helped us to spread this work by sharing with your friends or leaving us a review wherever you get your podcasts. That's really huge. Thanks also to our patrons on Patreon for helping us to make this podcast happen. If you'd like to donate to the cause you can find [email protected] slash down the wormhole podcast. And make sure you send in your questions for our new q&a segment as well. So hit us up on Facebook, Twitter or through our website at down the wormhole.com
No comments yet. Be the first to say something!